The manufacturing industry is undergoing a major transformation, fueled by rapid advancements in artificial intelligence (AI) and the rise of generative AI (GenAI). Early applications of AI and GenAI are helping manufacturers achieve significant gains in productivity, quality, and efficiency. This comes at a critical time as the industry faces skilled labor shortages, supply chain disruptions, and a highly competitive global market.
However, the integration of AI also introduces challenges that manufacturers must navigate carefully to fully reap the benefits of these innovations.
AI is addressing key industry challenges through manufacturing-specific solutions that benefit manufacturers, their workforce, and their customers. Here’s how:
1. Innovative Product Design
Generative AI is enhancing human-led product design by accelerating innovation and creating a cycle of market leadership through competitive advantage. AI and machine learning (ML) streamline the design process by automating tasks to increase efficiency and output. By analyzing previous designs, generating new concepts, and testing prototypes, AI helps engineers adopt more agile, rapid design practices.
Challenge: Adopting AI for product design requires a cultural shift and investment in reskilling the workforce, which can be difficult for companies with long-established processes.
2. Process Optimization
AI and GenAI excel at optimizing production processes, bridging the gap between the physical and digital worlds through real-time monitoring and control. This enables manufacturers to enhance quality, efficiency, and cost-effectiveness. AI-powered systems can proactively detect product defects and anomalies, allowing early corrections and minimizing waste. These systems can also optimize energy usage and reduce waste, contributing to sustainability efforts. The continuous, data-driven optimization by AI ensures ongoing process improvements based on real-time operational insights.
Challenge: Implementing AI for process optimization may require significant upfront investment in technology infrastructure, and some companies may struggle with integrating AI into legacy systems.
3. Supply Chain Management
AI enhances supply chain management by providing predictive insights that improve sourcing, production scheduling, logistics, and inventory management. By analyzing data from various points in the supply chain, AI facilitates smarter decision-making, resulting in greater efficiency and reduced costs. Generative AI can also enhance demand forecasting, using historical data to anticipate market fluctuations, while AI helps identify reliable suppliers based on factors like cost, quality, and dependability.
Challenge: Supply chains are complex, and using AI effectively requires access to high-quality, real-time data across all stages of the supply chain, which can be difficult to achieve. Additionally, AI-driven supply chains are more vulnerable to cybersecurity risks, as cyberattacks can disrupt AI systems and lead to widespread operational impacts.
4. Skill Gap Augmentation
While technology and processes are key to manufacturing, skilled workers are the backbone of the industry. However, the “Great Retirement” has led to a significant shortage of experienced workers with in-depth process knowledge. Generative AI can bridge this gap by offering training simulations and guidance to help new, less experienced workers reduce the learning curve, allowing them to quickly gain necessary expertise.
Challenge: While AI can augment the workforce, its adoption can also lead to resistance from workers fearing automation will replace their jobs. Managing this transition requires a balance between reskilling programs and workforce engagement.
5. Risk Management
Manufacturing operations are exposed to various risks, including cyber threats, operational hazards, and unexpected disruptions. Generative AI can help mitigate these risks by analyzing data to simulate potential scenarios and enabling manufacturers to create proactive contingency plans. This helps ensure better preparedness for disruptions before they occur.
Challenge: AI-driven risk management systems require extensive data to function effectively. Inconsistent or incomplete data can limit AI’s ability to predict risks accurately. Moreover, reliance on AI systems introduces new risks related to algorithmic biases and unforeseen system failures.
6. Data Privacy and Security
AI relies heavily on vast amounts of data, including proprietary company data, operational metrics, and personal information. This creates a challenge for manufacturers, as they need to ensure that data privacy laws and security protocols are strictly adhered to, preventing breaches and maintaining customer trust.
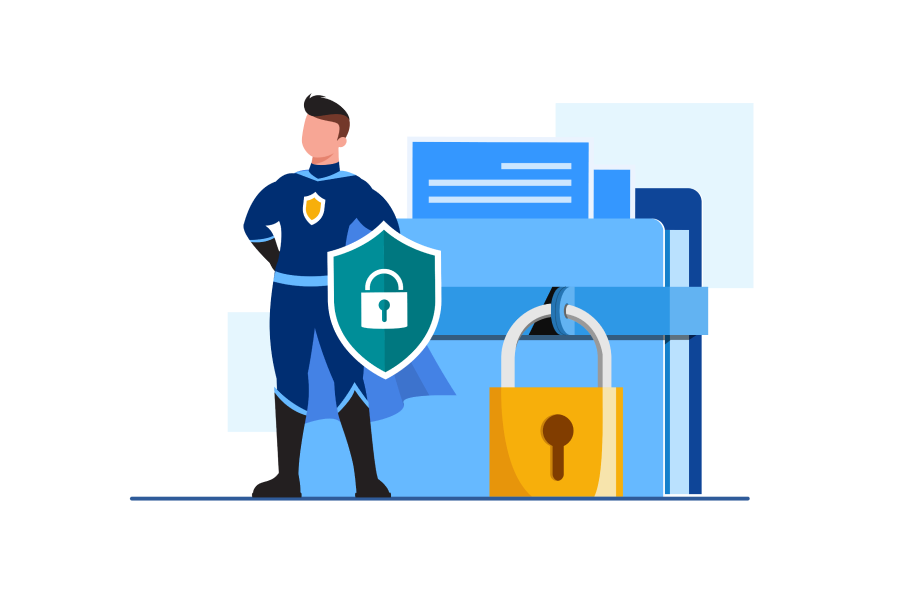
Challenge: Data security is a critical concern, especially as AI systems are increasingly targeted by hackers. Manufacturers must invest in strong cybersecurity measures to protect their AI systems from malicious attacks and ensure compliance with data protection regulations such as GDPR.
7. Ethical AI Use and Regulatory Compliance
The deployment of AI in manufacturing also raises ethical concerns, such as the potential for biased decision-making and the impact on jobs. Additionally, regulatory frameworks surrounding AI use in manufacturing are still evolving, meaning businesses may face uncertainty about compliance with future laws.
Challenge: Companies need to proactively develop internal AI ethics policies, ensure their systems are free from biases, and stay updated on the changing regulatory landscape to avoid future legal issues.
How the future of manufacturing is being transformed
AI and generative AI are ushering in a new era of innovation for the manufacturing industry, with the potential to dramatically reshape its future. To fully capitalize on the transformative power of AI and generative AI, manufacturers must first establish the right storage infrastructure—a crucial but often overlooked step in the AI deployment process. Proper storage solutions are essential to ensure the seamless operation of AI systems, especially during large-scale data processing.
Many manufacturers today rely on outdated IT systems and traditional data storage models that are ill-equipped for AI’s demands. Without modern storage solutions, AI processes may stall or fail to deliver optimal results. Advanced storage technologies, such as distributed storage systems, data compression, and efficient data indexing, are critical to achieving the speed, scale, and flexibility required for AI-powered manufacturing.
If we look back to the pre-Industrial Revolution era—roughly 200 years ago—life was completely different, with families working manually on farms to meet their needs. Fast forward another 200 years from now, and life is likely to be radically transformed, with disruptive technologies like AI and generative AI leading the charge. While the specifics of the future are still unclear, one thing is certain: the innovations brought by AI will revolutionize manufacturing, paving the way for new ideas, processes, and progress that will ultimately reshape the world.
These technologies won’t just improve efficiency—they will redefine what is possible in manufacturing, marking the beginning of a new era of industrial progress. The future of manufacturing will be built on the innovations and breakthroughs made today, creating a lasting impact on humanity’s advancement.